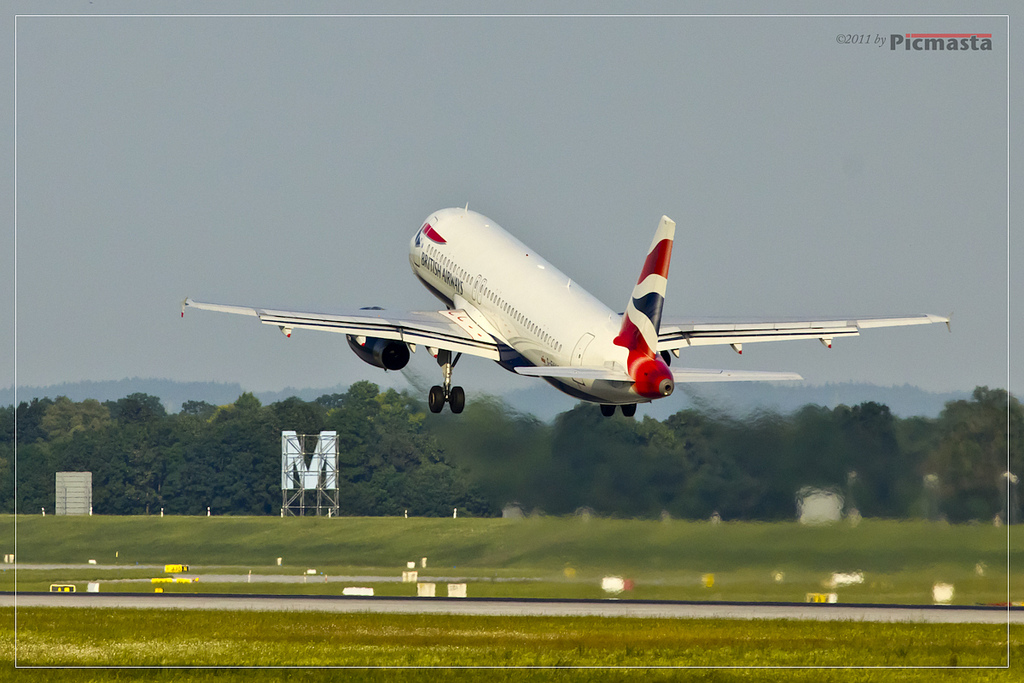
This post is also available in:
עברית (Hebrew)
The 9/11 attacks are among the most well-known security threats, and they upended how we travel. To protect passengers and crews, airports have made finding dangerous items their primary objective. Recognizing dangerous behaviors with predictive analytics and machine learning complement current security measures and promotes better flying conditions.
The theory behind behavior recognition is based on the theory that when someone is in the process of carrying out a criminal or terrorist act, that person exhibits behavior that is out of the norm. Sensors, video data, and other technologies supply the key to behavior recognition, identifying nervousness, stress, etc.
According to datanami.com, this type of behavior is often a tip-off that something is wrong, and can be split into two categories – micro behavior and macro behavior. Facial expressions, perspiration, lack of eye contact are micro examples. Macro behavior is broader movement throughout the space, such as attempting to hide his or her face by turning away when someone approaches or trying to stay out of sight.
While currently, humans are responsible for behavior detection, detecting these behaviors could be more accurate if it was automated with technology. Basing on new analytics tools, airports can now build a 360-degree view of each person over a long period of time. By applying predictive analytics and reviewing these large sets of structured and unstructured data, supported by mapping tools, geolocation information and more, airport security would grade each person on their risk potential.
Ironically, technology is often better than humans at recognizing atypical human behavior. The most difficult feat for staff is tracking all the acts that combine to terrorist behaviors. Each of these acts on its own may not raise suspicion. It’s only when we can see their behavior end-to-end that we can get a complete view.
While threats can come from outside sources such as terrorists or passengers, they can also be instigated from within, planted by a disgruntled airport employee or contract worker. By using predictive analytics, security operation managers can monitor both access and behavior of internal employees and contractors, identifying dangerous insiders and halting an attack before it happens.
The security team should know which airport employees have access to assets, including physical ones (luggage, airplanes) and cyber information used in daily operations. By gathering big data and using predictive analytics, security can automatically monitor and note irregular employee movements.
Machine learning platforms can integrate data from various sources regarding employees, such as history of performance, criminal records, information system access including on-site VPN (virtual private network) usage, and physical movement within the airport terminals – data received from badge scans or IoT-based door locks and geo-spatial scanners.
Like other police departments in the United States, the National Gendarmerie in France is adding data analytics to its armory of crime fighting weapons. They are not abandoning proven techniques, but modernizing the force.